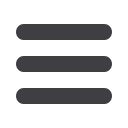
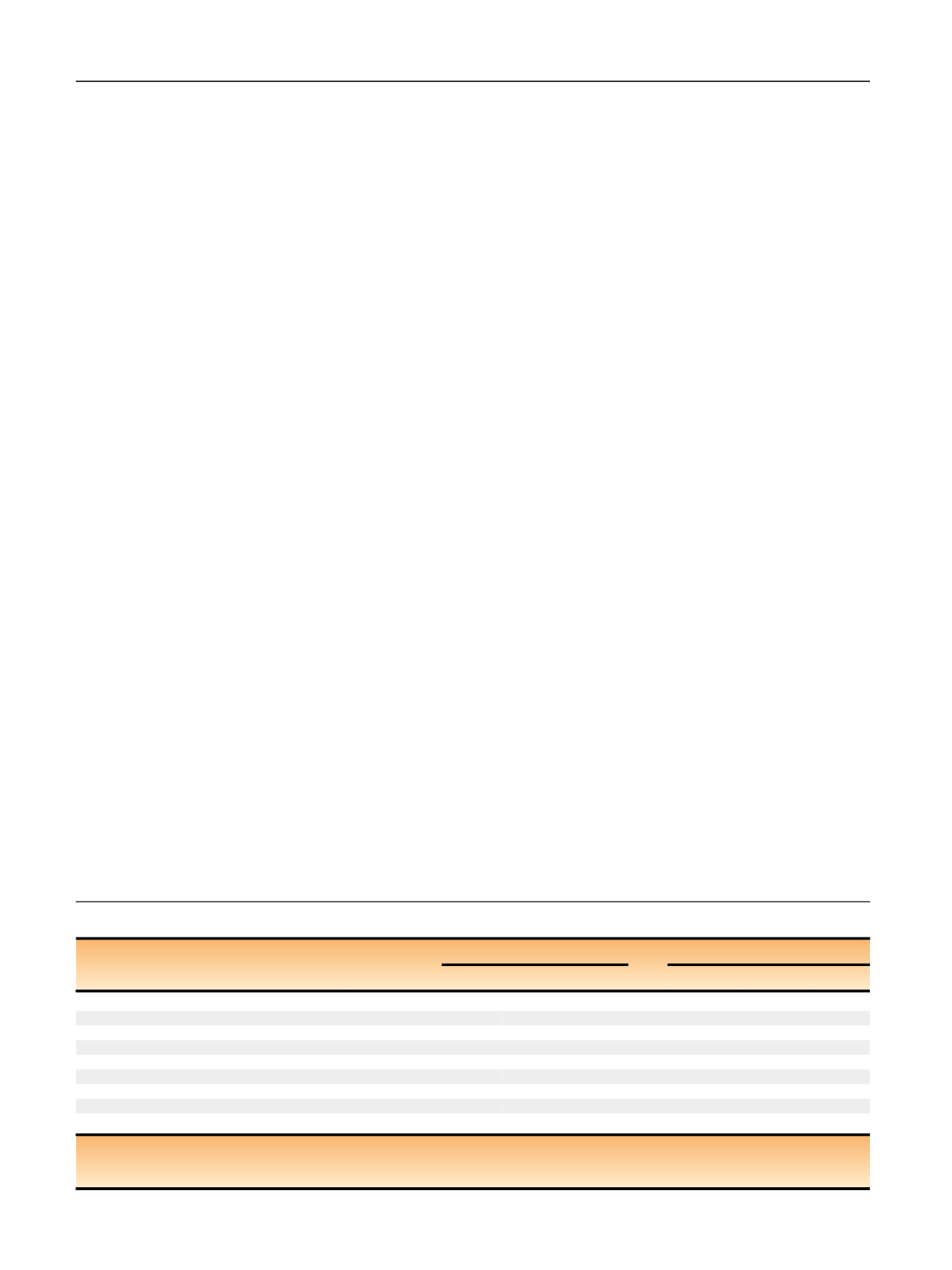
for each contributing dataset assuming a log-additive (trend) for the
effect of the telomere length-associated variants on RCC risk. Covariate
adjustment differed by dataset and are as follows: 19 signi
fi
cant
eigenvectors for IARC, age, and two signi
fi
cant eigenvectors for MD
Anderson Cancer Center, study indicator variables for NCI1, sex, and
three signi
fi
cant eigenvectors for NCI2, and no covariate adjustment for
the UK study. RCC association results for telomere length-associated
variants fromeach dataset were combined bymeta-analysis using a
fi
xed
effects model. Cochran
’
s Q tests for heterogeneity were conducted to
identify a lack of consistency across studies.
A GRS was calculated for the nine telomere length-associated
variants as follows:
GRS
i
¼
X
9
j
¼
1
w
j
x
ij
where GRS
i
is the risk score for individual
i
,
x
ij
is the number of telomere
length increasing alleles for the
j
th telomere length associated variant
and
w
j
is the weight or effect coef
fi
cient for each telomere length
associated variant. A higher GRS value for an individual indicates
longer genetically inferred telomere length. Previously published
telomere length associated effect estimates (
b
values) scaled to
estimated kilobases of telomere length per length increasing allele
were used for
w
j
[22 – 24]. GRS association tests were conducted
separately for each contributing study using the same covariates as
the single SNP association tests previously described. Results from
each study were merged by
fi
xed effects meta-analysis and hetero-
geneity tests were conducted to detect potential departures from
homogeneity. Additionally, subanalyses by RCC subtype as well as
analyses strati
fi
ed by sex, body mass index, history of hypertension,
and smoking status were conducted to comprehensively assess the
relationship between telomere length-associated variants and RCC
risk.
In addition to the GRS analysis, summary statistics from the nine
telomere length-associated variants were also combined in analyses using
an inverse variance weighting method and a likelihood-based method
[26] .Both methods use average summary association estimates for the
telomere length-associated variants with RCC risk to estimate the overall
effect of telomere length on RCC risk. These methods produce similar
estimates and precision as individual-level data, but have the advantage of
using effect statistics from different studies. An online web tool by
Burgess et al
[26]accessed at
https://sb452.shinyapps.io/summarized/on
February 10, 2017 was used to calculate the inverse variance and
likelihood-based estimates. Tests of heterogeneity were performed to
assess if a telomere length associated variant
’
s effect on RCC is
proportional to its effect on telomere length. Additionally, MR-Egger
regression models were
fi
t to evaluate the potential for pleiotropic effects
of variants
[27].
Unless otherwise stated, statistical analyses and plotting
were performed on a 64-bit build of R version 3.3.0
“
Supposedly
Educational
”
. Meta-analyses were performed using the R package
metafor
and Egger regression
[27]was performed using the R package
MendelianRandomization
. All statistical tests were two-sided with
p
values less than 0.05 considered signi
fi
cant.
3.
Results
Associations between the telomere length-associated var-
iants and RCC risk are reported in
Table 1and Supplemen-
tary Figure 1. Of the nine telomere length-associated
variants, five variants (rs10936599, rs2736100, rs9420907,
rs8105767, and rs6772228) displayed evidence for an
individual association with RCC risk (
p
<
0.05) and three
(rs10936599, rs2736100, rs9420907) were associated at
Bonferroni corrected levels (
p
<
0.006). This is substantially
more than the number of telomere length variants
associated with RCC risk that would be expected by chance
(exact binomial
p
<
0.0001). For all the telomere length-
related variants associated with RCC, the allele related to
longer telomere length was associated with an increased
risk of RCC. There was no evidence for heterogeneity in
effect estimates across studies.
We observed a highly statistically significant association
between the telomere length GRS and RCC risk (odds ratio
[OR] = 2.07 per predicted kilobase increase, 95% confidence
interval [CI] = 1.70
–
2.53,
p
<
0.0001;
Fig. 1), indicating
longer genetically inferred telomere length is associated
with increased RCC risk. In an analysis of GRS deciles, a
generally monotonic trend across deciles was observed
( Fig. 2). After removing two telomere length variants from
the GRS that were in linkage disequilibrium (LD) with RCC
susceptibility loci reported in the RCC GWAS (rs10936599 in
LD with rs10936602, and rs9420907 in LD with rs11813268;
R
2
0.59 and 0.76 in the CEU 1000 Genomes population,
Table 1
–
Associations of telomere length associated variants with renal cell carcinoma (RCC) risk
Nearby gene
Chr
Position
SNP
Telomere length association
Association with RCC risk
Allele
s aBet
a bS
E bBeta
SE
p
value
ACYP2
2
54329370
rs11125529
C/A
0.0669
0.0119
0.0143
0.0270
0.6
PXK
3
58376019
rs6772228
A/T
0.1200
0.0191
0.1198
0.0481
0.01
TERC
3
170974795
rs10936599
T/C
0.1173
0.0097
0.1001
0.0220
<
0.0001
NAF1
4
164227270
rs7675998
A/G
0.0897
0.0109
0.0133
0.0226
0.5
TERT
5
1339516
rs2736100
A/C
0.0942
0.0109
0.0678
0.0187
0.0003
OBFC1
10
105666455
rs9420907
A/C
0.0828
0.0120
0.1165
0.0264
<
0.0001
CTC1
17
8136092
rs3027234
T/C
0.0573
0.0110
0.0159
0.0228
0.5
ZNF208
19
22007281
rs8105767
A/G
0.0576
0.0096
0.0479
0.0207
0.02
RTEL1
20
61892066
rs755017
A/G
0.0741
0.0131
0.0117
0.0289
0.7
Chr = chromosome; SE = standard error; SNP = single nucleotide polymorphism.
a
Alleles are short allele/long allele. Short alleles are used as the reference in both the telomere length and RCC association models.
b
Beta and standard error estimates are from published association studies on leukocyte telomere length
[22 – 24].
E U R O P E A N U R O L O GY 7 2 ( 2 0 17 ) 74 7
–
7 5 4
750